Bridging the gap: A comparative analysis of traditional and neural network regression methods for predicting university entrant performance in SUAST examination
DOI:
https://doi.org/10.59120/drj.v14i2.116Keywords:
Academic performance, multiple linear regression, multi-layer perceptron neural network, predictive modeling, state university aptitude and scholarship testAbstract
In developing countries like the Philippines, access to free and high-quality tertiary education is crucial for better job opportunities. The State University Aptitude and Scholarship Test (SUAST) is used as a college admission examination by Davao Oriental State University (DOrSU). However, the passing rate for SUAST was only 54% for the academic year 2018-2023, and non-passers were still accepted due to policy changes, which undermine the purpose of the examination. This study aimed to identify the factors that influence the performance of university entrants in the SUAST examination using a researcher-made survey questionnaire administered online, utilizing both multiple-layer perceptron neural network (MLPNN) and multiple linear regression analysis (MLR) methods. A sample size of 359 was recommended, and the study found that family income, senior high school general weighted average (SHSGWA), library entry, intrinsic goal, openness and intellect, and behavioral reaction were significant predictors of SUAST exam scores. MLPNN analysis further identified library access and resources, family income, and academic self-belief as the most important predictors of SUAST exam scores, and MLPNN outperformed MLR. This study provides recommendations for DepEd and HEI’s to enhance the preparation and performance of students taking the SUAST exam, such as offering study materials and test-taking strategies, evaluating alternative admission tests, and reviewing the content validity of the questionnaire.
Downloads
References
Abbasi, N., and Ghosh, S. (2020). Construction and standardization of examination anxiety scale for adolescent students. International Journal of Assessment Tools in Education, 7(4), 522-534.
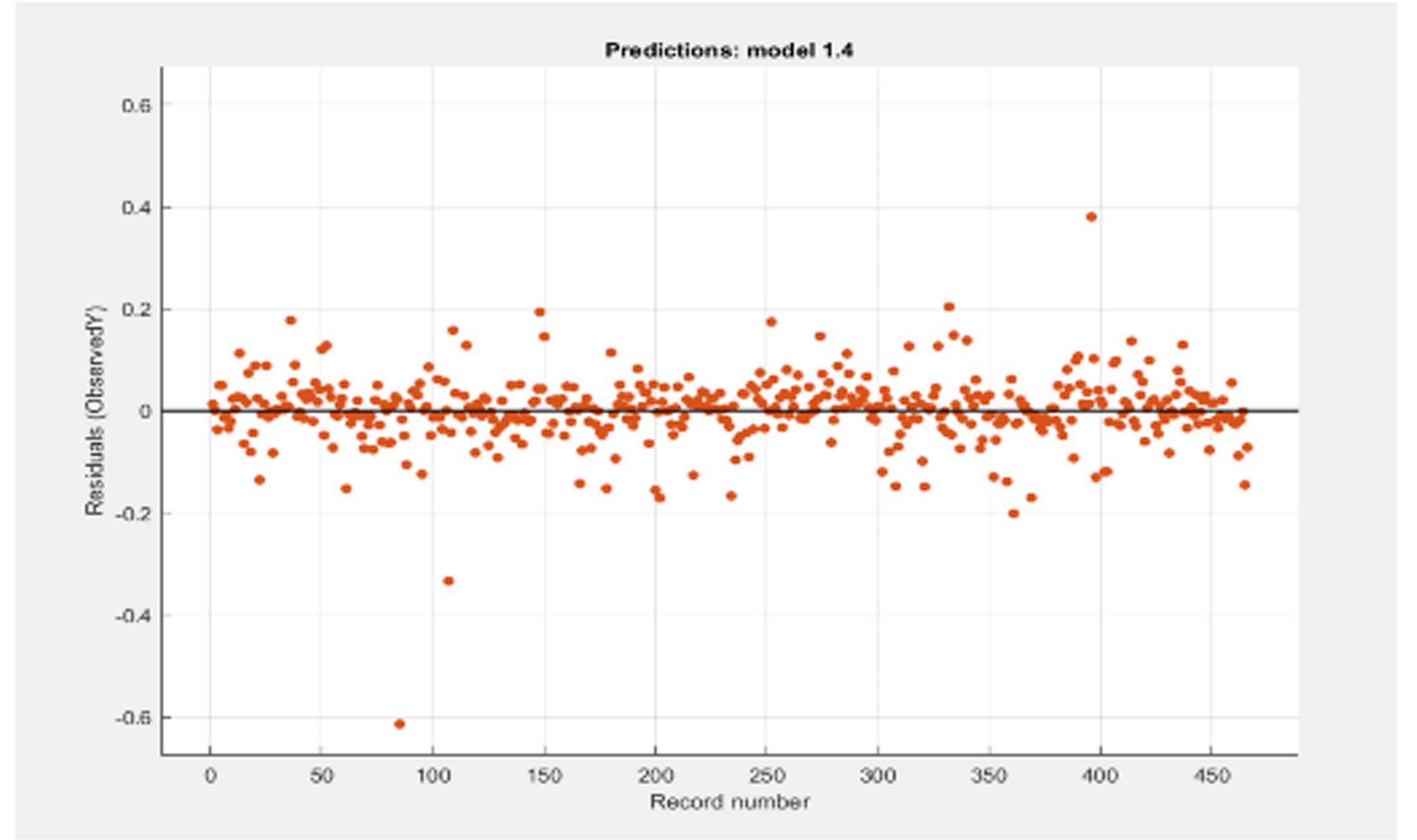
Downloads
Published
Issue
Section
License
Copyright (c) 2023 Nikka A. Singh, Diether C. Montejo

This work is licensed under a Creative Commons Attribution-NonCommercial 4.0 International License.
DRJ is an open-access journal and the article's license is CC-BY-NC. This license allows others to distribute, remix, tweak, and build on the author's work, as long as they give credit to the original work. Authors retain the copyright and grant the journal/publisher non-exclusive publishing rights with the work simultaneously licensed under a https://creativecommons.org/licenses/by-nc/4.0/.